Abstract:
This talk presents the transition from statistical/deep clustering to causality learning, a significant challenge in unravelling variable relationships through data. As an effective approach for structuring data, deep clustering works by learning the deep representation features to get the clustering probability distributions. Although, there can be a lot of important knowledge including clusters, outliers, and causalities hidden in the learned distributions, it is hard to infer and visually present the hidden knowledge. To solve the above problem, we propose a new framework, Hamiltonian-clustering Modernized Asymmetric Causality (HMAC), which is a combination of Hamiltonian cycle and deep clustering, embarking on a novel exploration of modernized causality learning.
HMAC consists of three core advancements -- 1) integrating global and local data structures of data in a new deep clustering algorithm, GLDC; 2) learning the indirect causalities, i.e., the potential extended relationships between different clusters representing subjects, and the direct causalities, i.e., the change of some specific features when modifying the value of another feature in different clusters, by Generalized Measures of Correlation (GMC) which deals with asymmetries to infer the causalities from data; 3) mapping the learned clusters, outliers, and causalities by the optimal Hamiltonian cycle of the GMCs between different clusters in a Radviz-type visualization. HMAC can be utilized for causality learning in various fields, such as economics and biomedicine. The theoretical analysis and experiments illustrate the superiority of HMAC. Joint work with Tianyi Huang and Shenghui Cheng.
About the Speaker:
张正军教授现为中国科公司大学经济与管理公司长聘教授和统计与数据科学系系主任,中国科公司预测科学研究中心副主任,原美国威斯康辛大学统计系终身教授和系副主任,威斯康辛大员工物医学信息系兼职教授,国际数理统计协会执行委员和财务总监(July 2016 -- July 2022),国际数理统计协会会士,美国统计协会会士。现担任JASA,JBES, Statistica Sinica, JDS, EJS、STaRF等国际期刊副主编。主要研究方向包括统计理论和方法、计量经济学、金融计量学、计算医学与实践、 极端气候等等。在国际顶级期刊:统计(AoS,JASA,JRSSB)、计量(JoE, EE)、金融(JBES, JBF)、医学(AFM, Vaccines,npj Precision Oncology)、气象 (ATM) 等发表论文上百篇。代表性工作和首创性思想和作品包括: 新极值理论、绝对和相对同步有效性(AbRelaTEs)、双边截断极值惩罚变量选择机器学习模型(TWT-LR-ETP)、商相关系数(QCC、TQCC)、非对称广义相关系数(GMC)、滞后尾部相依系数(lambda_k)、最大线性回归模型(MaxLR)、最大逻辑回归模型(Max-logistic)、EGB2期权定价公式、盯市在险价值(MMVaR)、条件极值Frechet自回归(AcF), 虚拟标准数字货币(VSTC),新冠基因组学、癌症基因组学的几何空间(DARPA: Mathematical Challenge Fifteen: The Geometry of Genome Space),等等。
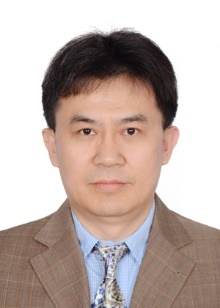